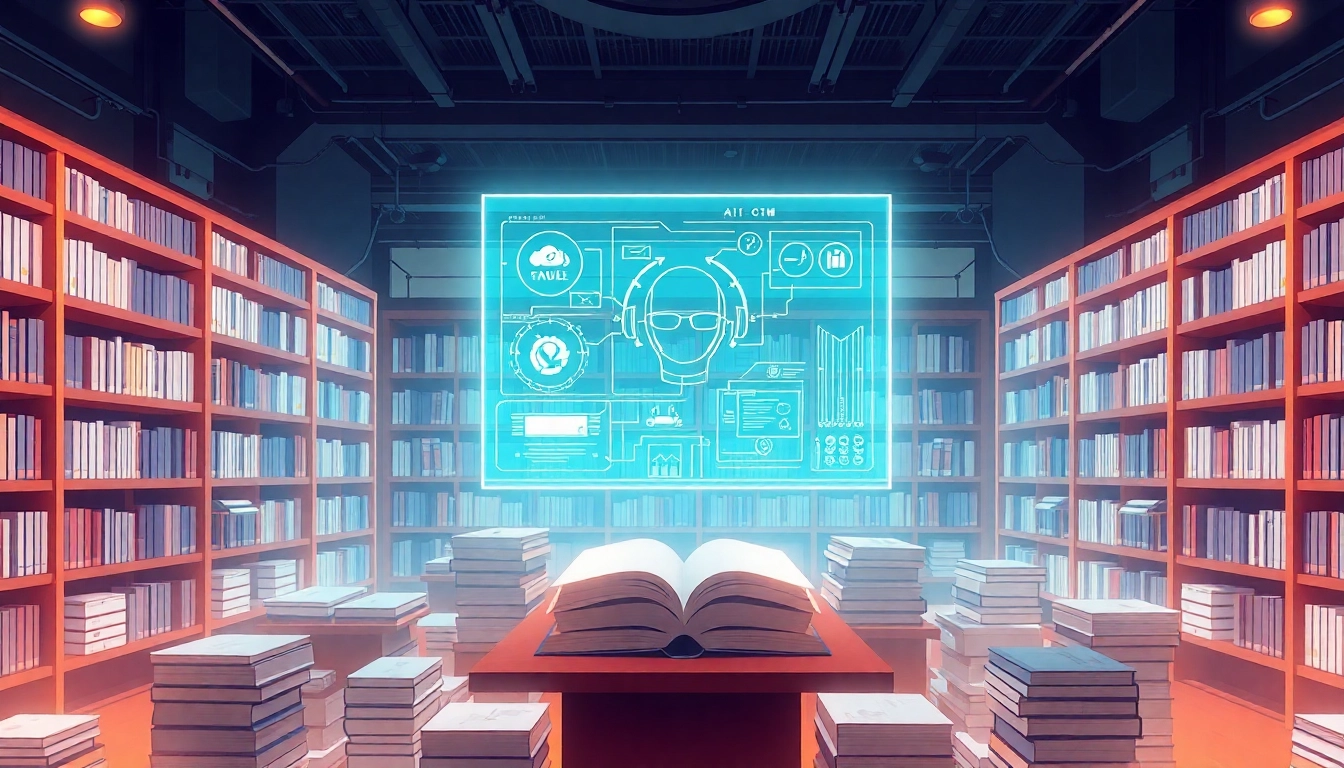
Understanding AI Opinion Search
What is AI Opinion Search?
AI Opinion Search represents a fusion of artificial intelligence and information retrieval, designed to streamline the process of gathering opinions on various topics across extensive datasets. Unlike traditional search engines, which primarily rely on keyword matching, AI opinion search employs advanced algorithms to interpret and contextualize data, offering users nuanced insights into prevailing sentiments and expert opinions. This empowers researchers and decision-makers to navigate vast amounts of information efficiently, making informed choices based on a comprehensive view of public discourse and scholarly insights.
With tools that enhance ease of access, such as AI Opinion search, users can delve deeper into specific topics, extract relevant insights, and enhance their understanding in an ever-evolving data landscape.
Benefits of Using AI for Opinion Research
The utilization of AI in opinion research provides numerous advantages that can significantly enhance both the quality and speed of research outcomes. Here are some key benefits:
- Efficiency: AI algorithms analyze huge volumes of data in real-time, drastically reducing the time required for traditional research methods.
- Accuracy: By leveraging machine learning techniques, AI can offer more precise insights than traditional analytic methods, minimizing human error.
- Contextual Understanding: AI tools can understand the context surrounding opinions and sentiments, aiding researchers in identifying underlying trends.
- Scalability: As data grows exponentially, AI systems can scale operations to accommodate more extensive datasets without a proportional increase in resources.
- Enhanced Decision-Making: Access to insights generated by AI can lead to more informed and strategic decision-making processes.
Key Differences between Traditional and AI Opinion Search
Understanding the distinctions between traditional and AI-powered opinion search methods is essential for leveraging their respective strengths effectively. Below are the key differences:
- Data Processing: Traditional methods often involve manual data collection and analysis, which can be time-consuming and subjective. In contrast, AI opinion search automates these processes using intelligent algorithms.
- Output Type: While traditional searches yield results based solely on keywords, AI opinion search provides a more interpretive output, including sentiment analysis and relevance rankings.
- User Interaction: AI systems adapt to user queries and behavior, learning and refining the search results over time, as opposed to static traditional search algorithms.
- Integration and Adaptability: AI platforms can integrate with various data sources and adapt to changing trends and user needs more readily than traditional systems.
How AI Opinion Search Works
Algorithms Powering AI Opinion Search
The backbone of AI Opinion Search lies in sophisticated algorithms that enable effective data processing and extraction of valuable insights. These algorithms typically include:
- Natural Language Processing (NLP): NLP enables AI systems to understand human language nuances, allowing them to interpret sentiments and opinions present in text data.
- Machine Learning: Research models update and improve over time by learning from new data, refining their analysis and prediction accuracy.
- Semantic Analysis: This technique focuses on understanding the meaning behind the words, ensuring that the search results are contextually appropriate and relevant.
- Sentiment Analysis: Leveraging statistical models, AI can ascertain whether opinions expressed in data are positive, negative, or neutral, providing quick insights into public sentiment.
Data Sources Utilized in AI Opinion Systems
AI opinion search engines tap into diverse data sources to improve the richness and accuracy of their insights. These sources can include:
- Academic Journals: Scholarly articles and studies provide a repository of verified opinions and research analysis.
- Social Media: User-generated content from platforms like Twitter, Facebook, and LinkedIn can highlight public sentiment and trending topics.
- News Outlets: Articles and editorials offer a wealth of opinions on current events, reflecting diverse perspectives.
- Surveys and Polls: Aggregated data from surveys can provide quantifiable insights into public opinion.
- Blogs and Forums: These informal platforms often reveal grassroots opinions that formal sources might overlook.
User Interaction and Experience with AI Opinion Search
User interaction is a crucial aspect of AI opinion search. The experience typically involves:
- Personalized Queries: Users can tailor their searches based on specific interests or questions, receiving targeted results.
- Visual Data Presentation: Infographics and visual data representations make it easier to digest complex information.
- Real-time Insights: AI systems provide updates as new data becomes available, ensuring that users have access to the most current information.
- Feedback Mechanisms: Users can provide feedback on search results, allowing the AI to learn and refine its accuracy over time.
Implementing AI Opinion Search in Your Research Strategy
Identifying Research Needs with AI Opinion Search
Before diving into AI opinion search, it is essential to identify your specific research needs, which include:
- Defining Objectives: Clearly outline what you wish to achieve—whether it is understanding public sentiment, identifying gaps in existing research, or exploring competitive landscapes.
- Target Audience Identification: Recognize who will benefit from the research findings and tailor the search accordingly.
- Key Topics and Questions: Determine the critical areas of focus and formulate precise questions that will guide your search.
- Evaluation Criteria: Establish criteria for assessing the relevance and quality of information retrieved from AI sources.
Best Practices for Integrating AI Opinion Search
To maximize the impact of AI opinion search in your research strategy, consider adopting the following best practices:
- Combine AI with Traditional Methods: Use AI tools to complement traditional research methods, creating a more robust analysis.
- Regular Updates: Frequently update your search queries based on emerging trends and new data to maintain relevance.
- Cross-Verification: Validate AI-generated insights against established sources to ensure reliability.
- Engage Diverse Sources: Tap into various data sources to obtain a well-rounded understanding of opinions and sentiments around your topic.
Case Studies of Successful AI Opinion Implementation
Examining real-world applications of AI opinion search can provide valuable insights and inspiration. Here are a few notable case studies:
- Case Study 1: Academic Research – A leading university employed AI-driven search tools to analyze dissertations and academic papers in sociology. The result: significantly improved research quality and quicker identification of relevant literature.
- Case Study 2: Market Analysis – A major retail brand used AI to analyze consumer opinions from social media. By understanding the sentiment around their products, they enhanced their marketing strategies and product development.
- Case Study 3: Political Polling – During an election, a political campaign implemented AI opinion search to track voter sentiment in real-time, allowing for rapid adjustments in campaign messaging.
Challenges and Limitations of AI Opinion Search
Ethical Considerations in AI Opinion Research
As with any technology, ethical considerations play a crucial role in AI opinion research. Researchers must grapple with issues such as data privacy, consent, and transparency. Using AI to manipulate public opinion or disregarding individual privacy rights can have significant consequences. Therefore, adhering to ethical guidelines and maintaining a focus on responsible AI usage is paramount.
Addressing Bias in AI Opinion Search Results
Bias in AI algorithms can skew results, leading to misleading conclusions. Some steps to mitigate bias include:
- Data Diversity: Ensure datasets encompass various demographics and perspectives to avoid skewed results.
- Regular Audits: Conduct frequent reviews of algorithm performance to identify and rectify any inherent biases.
- Transparency: Maintain openness about how algorithms function and the data used to train them.
Technical Limitations and Future Developments
The realm of AI opinion search is evolving, yet it faces certain technical limitations, including:
- Complexity in Contextual Interpretation: While AI excels in data processing, understanding nuanced contexts can still pose challenges.
- Resource Intensive: Developing and maintaining robust AI systems can be resource-heavy, requiring significant investment in technology and expertise.
- Dependence on Quality Data: The effectiveness of AI opinion systems is contingent on the quality of input data; poor data quality can lead to unreliable results.
Future developments focused on improving natural language understanding and reducing biases will propel AI opinion search into even more dynamic territories.
The Future of AI Opinion Search in Various Industries
AI Opinion Search in Academia and Research
In academia, AI opinion search will continue to transform research methodologies, enhancing access to relevant literature and enabling deeper insights into complex issues. The integration of AI tools into research practices fosters collaboration and the sharing of diverse viewpoints, which is essential for innovative breakthroughs.
Applications in Business Decision-Making
Businesses are leveraging AI opinion search to guide strategic options, enhance consumer insights, and anticipate market trends. For example, companies can analyze customer feedback and sentiment to refine product offerings and realize competitive advantages.
Potential in Legal and Policy Research
AI opinion search applications in legal and policy realms can accelerate decision-making processes by synthesizing vast quantities of case law and public opinion data. This allows policymakers and legal professionals to base their decisions on comprehensive analyses rather than piecemeal information.